What is Geospatial Data Science?
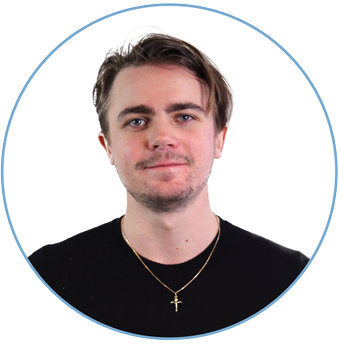
Michael Tuijp
Geospatial Data ScientistData Science is a buzzword. It is used as a synonym for (Big) Data Analysis, Machine Learning, Deep Learning and Artificial Intelligence. Because of the broad use of the term there seems to be confusion about what Geospatial Data Science actually is (and what it is not). In a series of three blogs that I will be publish these coming weeks, I will shed light on the terminology and possibilities of this phenomenon. In this first blog, I start at the beginning: what is Geospatial Data Science?
The Geospatial Data Science lifecycle
A Geospatial Data Science (GDS) process can be described in seven stages:
- Defining the business goal;
- Data mining (Data Engineering);
- Data cleaning (Data Engineering);
- Data exploration (Data Analysis);
- Feature Engineering (Data Analysis);
- Predictive modelling (GDS/AI/ML/DL);
- Data Visualisation.
Image 1: the seven stages of (Geospatial) Data Science. Text continues below the image.
Stage 1: Defining the business goal
The first step is universal for any project involving data. Define the purpose. What do you want to know and why? Geospatial Data Science business is not an end in itself, but a means to make data-driven decisions.
As a Consultant, this is where I can add value for Tensing clients. Just mastering the technical side is not enough. As an external force, I can look at your organisation and your goals with an open mind. I can help you properly separate goals from resources. Besides, very often much more is possible than you think.
Stages 2 and 3: Data Engineering
Data engineering is the first technical step in all data-related processes. Step one of the Data Engineering-phase is collecting the data needed for the specific project. There is often a lot involved in unlocking the right data from the right sources and in the right formats. Therefore, look very critically at what you need and especially what you don't need. This greatly benefits efficiency later in the process.
After compiling a suitable dataset, it is time to clean up data. In practice, values are always missing, different tables exist that mean the same thing and inconsistency is often present.
Tensing primarily uses FME (an ETL tool optimised to work with geodata) to complete the Data Engineering phase. As a Geospatial Data Scientist, I can rely on 60 certified colleagues who are fully specialised in Geospatial Data Engineering.
Picture 2: FME is the best software choice in the field of Geospatial Data Engineering as far as Tensing is concerned. Text continues below the image.
Stage 4 and 5: Data Analysis
After completing the Data Engineering phase, it's time to start working with the data. Based on hypotheses, you test whether you can extract all the desired insights from the available dataset. You can do this using test visualisations. When you determine that your data selection is complete, you can continue to the next stage. Sometimes it happens that your Data Engineering steps need some fine-tuning before you go any further.
During the Feature Engineering step, you create new features based on existing data. These are values that are relevant to your model but are not included as separate variables. I'll take gross profit as an example: sales - purchase value. If you need profit as a separate feature to generate your predictive model, include it in your dataset during the Feature Engineering phase.
Executing the first five steps carefully is essential before you start the predictive modelling phase. First, because you will be faced with large lists of errors. Second, because the result will fall under the heading garbage in, garbage out. The insights that come out of your model are likely to show extreme outliers, may be way too positive (or negative), or they are just incompatible with reality.
Stage 6: Predictive modelling
Data science is mostly associated with the predictive modelling stage. Predicting trends based on past (and present) data. Artificial Intelligence, Machine Learning and Deep Learning are tools that enable predictive modelling. In a subsequent blog, I will elaborate on a practical example: predictive maintenance. So keep an eye on our social channels and website!
Predictive modelling is impossible without carefully going through the previous steps. Data has value only if it is 100% correct. That fact is an absolute truth when practising Data Science.
Stage 7: Data visualisation
Data visualisation is what you (usually) do it all for. To show certain insights to a wide range of stakeholders in an understandable way is a dashboard, a heatmap or a 3D visualisation, whichever suits your project best.
The geographical component
The world of geographic information systems has carved its own niche. Working with location data is just that little bit different. This also applies to Geospatial Data Science. Properly integrating data sources without a geographical component with geometric data is the main challenge. Extensive knowledge of geographic data is absolutely necessary to create predictive models that can tell you not only what is going to happen, but also where.
Need help with a Geospatial Data Science challenge? Feel free to contact me!